Most likely too questions, also referred to as MLQs, are a specialized form of question employed in the context of information retrieval. MLQs are designed to identify the most probable correct answer to a user’s query based on available data. They leverage statistical models and machine learning algorithms to estimate the likelihood of each potential response being the most relevant and accurate one. By harnessing the power of statistical analysis and predictive modeling, MLQs enhance the effectiveness of search engines and other information retrieval systems.
The significance of MLQs lies in their ability to refine search results, leading to more precise and efficient retrieval of information. By prioritizing the most likely correct answer, MLQs minimize the need for users to sift through irrelevant or incorrect responses. This not only saves time and effort but also improves the overall user experience, enhancing satisfaction and productivity.
Moving forward, we will delve into the intricate workings of MLQs, exploring the underlying statistical models and machine learning techniques that drive their functionality. We will also examine the historical evolution of MLQs, tracing their development from early theoretical concepts to their widespread adoption in modern search engines and information retrieval systems.
MOST LIKELY TOO QUESTIONS
Most likely too questions (MLQs) are a crucial component of modern information retrieval systems, enhancing the accuracy and efficiency of search results. They encompass various aspects, each playing a significant role in their functionality and impact on the user experience.
- Statistical Modeling: MLQs leverage statistical models to estimate the probability of each potential answer being the most relevant.
- Machine Learning Algorithms: These algorithms drive the statistical modeling process, enabling MLQs to learn from data and improve their accuracy over time.
- Information Retrieval: MLQs are employed in information retrieval systems to refine search results, prioritizing the most likely correct answer.
- User Experience: MLQs enhance the user experience by minimizing the need to sift through irrelevant or incorrect responses, saving time and effort.
- Efficiency: By prioritizing the most likely correct answer, MLQs improve the efficiency of information retrieval, leading to faster and more targeted results.
- Accuracy: The statistical modeling and machine learning algorithms employed in MLQs contribute to increased accuracy in identifying the most relevant answer.
- Historical Evolution: MLQs have evolved from theoretical concepts to widespread adoption in modern search engines and information retrieval systems.
- Example: A search engine using MLQs might prioritize the answer “Barack Obama” when a user queries “Who was the first African American president of the United States?”
- Relevance to Main Topic: MLQs are essential to the main topic of information retrieval, as they enhance the effectiveness and accuracy of search results.
In summary, these key aspects highlight the importance of MLQs in modern information retrieval systems. By leveraging statistical modeling and machine learning algorithms, MLQs refine search results, improve user experience, enhance efficiency, and contribute to the overall accuracy and relevance of the information retrieval process.
Statistical Modeling
Statistical modeling plays a pivotal role in the functioning of most likely too questions (MLQs). It provides the foundation for estimating the probability of each potential answer being the most relevant to a user’s query. This estimation process is crucial for prioritizing and ranking the search results, ensuring that the most likely correct answer is presented prominently.
-
Facet 1: Probability Estimation
Statistical models employed in MLQs utilize various techniques to estimate the probability of each potential answer. These techniques may involve analyzing historical data, considering user behavior patterns, and leveraging natural language processing to understand the context of the query. -
Facet 2: Relevance Scoring
Based on the probability estimations, MLQs assign relevance scores to each potential answer. These scores reflect the likelihood of each answer being the most relevant to the user’s query. Higher relevance scores indicate a greater probability of correctness. -
Facet 3: Result Ranking
The relevance scores assigned by MLQs are used to rank the search results. The potential answer with the highest relevance score is ranked first, followed by the remaining answers in descending order of their scores. This ranking helps users quickly identify the most likely correct answer. -
Facet 4: Continuous Learning
Statistical models used in MLQs are continuously refined and updated based on user feedback and interaction with the search engine. This ongoing learning process enables MLQs to improve their accuracy and relevance over time.
In summary, statistical modeling is the cornerstone of MLQs, enabling them to estimate the probability of each potential answer being the most relevant. Through probability estimation, relevance scoring, result ranking, and continuous learning, MLQs leverage statistical models to enhance the accuracy and effectiveness of information retrieval systems.
Machine Learning Algorithms
Machine learning algorithms are the driving force behind the statistical modeling process employed in most likely too questions (MLQs). These algorithms empower MLQs to continuously learn from data and refine their accuracy over time, resulting in more precise and reliable search results.
The integration of machine learning algorithms in MLQs offers several key advantages:
- Adaptability: Machine learning algorithms enable MLQs to adapt to changing search patterns and user behavior. By analyzing vast amounts of data, these algorithms can identify new trends and adjust their statistical models accordingly, ensuring that MLQs remain effective in providing relevant and up-to-date results.
- Accuracy Improvement: Over time, machine learning algorithms refine the statistical models used by MLQs, leading to improved accuracy in identifying the most likely correct answer. The algorithms analyze user feedback, search patterns, and other relevant data to identify areas where accuracy can be enhanced.
- Personalization: Machine learning algorithms allow MLQs to personalize search results based on user preferences and search history. By considering factors such as past queries, click-through rates, and dwell time, MLQs can tailor the ranking of search results to each individual user, providing a more relevant and personalized experience.
In summary, machine learning algorithms are essential components of MLQs, driving the statistical modeling process and enabling continuous learning and improvement. These algorithms enhance the adaptability, accuracy, and personalization of MLQs, contributing to their effectiveness in providing users with the most likely correct answers to their queries.
Information Retrieval
Most likely too questions (MLQs) play a pivotal role in modern information retrieval systems, enhancing the accuracy and efficiency of search results. Their integration into information retrieval systems brings forth several key benefits:
-
Facet 1: Relevance Enhancement
MLQs leverage statistical modeling and machine learning algorithms to prioritize the most relevant answers to user queries. By analyzing historical data, user behavior patterns, and the context of the query, MLQs can identify the most likely correct answer, improving the relevance and accuracy of search results.
-
Facet 2: Efficiency Improvement
MLQs enhance the efficiency of information retrieval by reducing the need for users to sift through irrelevant or incorrect responses. By prioritizing the most likely correct answer, MLQs save time and effort, leading to a more streamlined and productive user experience.
-
Facet 3: User Experience Optimization
The integration of MLQs into information retrieval systems optimizes the user experience by providing more relevant and accurate results. This reduces frustration and improves satisfaction, as users can quickly and easily find the information they seek.
-
Facet 4: Continuous Learning and Improvement
MLQs incorporate machine learning algorithms that enable continuous learning and improvement. By analyzing user feedback and interaction with the search engine, MLQs can refine their statistical models and enhance their accuracy over time, resulting in a consistent improvement in the quality of search results.
In summary, the integration of MLQs into information retrieval systems has revolutionized the way users access and interact with information. By prioritizing the most likely correct answer, MLQs enhance relevance, improve efficiency, optimize user experience, and facilitate continuous learning and improvement.
User Experience
In the realm of information retrieval, most likely too questions (MLQs) play a pivotal role in elevating the user experience. By prioritizing the most likely correct answer to a user’s query, MLQs significantly reduce the need for users to navigate through irrelevant or incorrect responses, ultimately saving them valuable time and effort.
-
Facet 1: Streamlined Search Results
MLQs leverage statistical modeling and machine learning algorithms to analyze vast amounts of data, identifying the most relevant answers to user queries. This refined approach eliminates the clutter of irrelevant information, presenting users with a concise and targeted list of results.
-
Facet 2: Enhanced Accuracy
The statistical models employed by MLQs are continuously trained and updated, ensuring high levels of accuracy in identifying the most likely correct answer. This reduces the likelihood of users encountering incorrect or misleading information, enhancing the overall credibility and reliability of the search results.
-
Facet 3: Time Savings
By prioritizing the most relevant answer, MLQs save users the time and effort required to manually sift through numerous search results. This streamlined approach allows users to quickly and efficiently find the information they seek, maximizing their productivity.
-
Facet 4: Reduced Frustration
The frustration often associated with navigating through irrelevant or incorrect search results is significantly reduced when using MLQs. By presenting users with a refined list of highly relevant answers, MLQs minimize the likelihood of confusion and frustration, enhancing the overall user experience.
In summary, the integration of MLQs into information retrieval systems profoundly enhances the user experience by streamlining search results, improving accuracy, saving time, and reducing frustration. These multifaceted benefits underscore the critical role of MLQs in shaping the future of information access and retrieval.
Efficiency
The efficiency of information retrieval is significantly enhanced by the prioritization of the most likely correct answer through most likely too questions (MLQs). This connection underscores the fundamental role of MLQs in streamlining the search process and delivering targeted results.
The effectiveness of MLQs stems from their ability to analyze vast amounts of data, leveraging statistical modeling and machine learning algorithms to identify the most relevant answer. By presenting this answer prominently, MLQs eliminate the need for users to manually sift through numerous search results, saving them valuable time and effort.
Consider a scenario where a user seeks information about the population of a particular country. Traditional search engines may return a multitude of results, including general overviews, historical data, and statistical reports. With MLQs, the most likely correct answer, such as the current population figure, is prioritized, allowing the user to access the desired information quickly and efficiently.
The practical significance of this efficiency is evident in various domains, including academic research, business intelligence, and everyday web browsing. For researchers, MLQs can expedite the process of finding relevant literature, enabling them to focus on analysis and interpretation rather than spending excessive time searching for information.
In conclusion, the efficiency gained through the prioritization of the most likely correct answer is a cornerstone of MLQs. This efficiency translates into faster and more targeted results, enhancing the overall productivity and user experience of information retrieval.
Accuracy
The accuracy of most likely too questions (MLQs) is directly linked to the statistical modeling and machine learning algorithms employed in their design. These algorithms analyze vast amounts of data, leveraging patterns and relationships to identify the most likely correct answer to a user’s query. The accuracy of MLQs has significant implications for information retrieval and user experience.
-
Facet 1: Statistical Modeling
Statistical modeling in MLQs involves analyzing historical data and user behavior patterns to estimate the probability of each potential answer being the most relevant. By considering factors such as query terms, document content, and user click-through rates, MLQs can assign relevance scores to each answer, with higher scores indicating a greater likelihood of correctness.
-
Facet 2: Machine Learning Algorithms
Machine learning algorithms play a crucial role in refining the statistical models used in MLQs. These algorithms identify patterns and relationships in the data that may not be immediately apparent to human analysts. By continuously learning and adapting, MLQs can improve their accuracy over time, leading to more precise and reliable results.
-
Facet 3: Real-World Examples
The accuracy of MLQs is evident in various real-world applications. For instance, search engines utilize MLQs to prioritize the most relevant web pages for a given query. Similarly, recommendation systems leverage MLQs to suggest personalized content to users based on their preferences and.
-
Facet 4: Implications for Users
The increased accuracy of MLQs has significant implications for users. By providing more precise and reliable results, MLQs save users time and effort in finding the information they seek. This enhanced accuracy also leads to greater user satisfaction and trust in the information retrieval process.
In conclusion, the statistical modeling and machine learning algorithms employed in MLQs contribute directly to their increased accuracy in identifying the most relevant answer. This accuracy is crucial for enhancing the overall effectiveness and user experience of information retrieval systems.
Historical Evolution
The historical evolution of most likely too questions (MLQs) is closely intertwined with the development of information retrieval systems. The concept of MLQs emerged from theoretical research in the field of information science, where researchers sought to develop methods for identifying the most relevant answer to a user’s query.
-
Facet 1: Early Theoretical Foundations
The theoretical foundations of MLQs can be traced back to the 1960s and 1970s, with researchers exploring statistical models and probabilistic approaches to information retrieval. These early theories laid the groundwork for the development of MLQs as a practical tool.
-
Facet 2: Integration into Search Engines
In the 1990s, MLQs began to be integrated into commercial search engines. The widespread adoption of MLQs by search engines such as Google and Bing marked a significant turning point in the evolution of information retrieval.
-
Facet 3: Machine Learning Advancements
The advent of machine learning algorithms in the 2000s further enhanced the capabilities of MLQs. Machine learning algorithms enabled MLQs to learn from user behavior and improve their accuracy over time.
-
Facet 4: Current Applications
Today, MLQs are an essential component of modern search engines and information retrieval systems. They are used to prioritize search results, recommend relevant content, and provide personalized experiences to users.
The historical evolution of MLQs highlights their continuous refinement and improvement over time. From their theoretical origins to their widespread adoption in practical applications, MLQs have played a pivotal role in shaping the way users access and interact with information.
Example
This example aptly illustrates the practical application of most likely too questions (MLQs) in modern information retrieval systems. When a user enters the query “Who was the first African American president of the United States?”, MLQs leverage statistical modeling and machine learning algorithms to analyze vast amounts of data, including historical records, biographical information, and user behavior patterns.
-
Facet 1: Relevance and Accuracy
MLQs assign relevance scores to each potential answer based on its likelihood of being the most relevant to the user’s query. In this case, the answer “Barack Obama” receives a high relevance score due to its factual accuracy and alignment with the query’s intent.
-
Facet 2: Efficiency and Time-Saving
By prioritizing the most likely correct answer, MLQs save users time and effort in manually sifting through numerous search results. This efficiency is particularly valuable in situations where users require quick and precise information.
-
Facet 3: Enhanced User Experience
The use of MLQs contributes to a positive user experience by providing users with the most relevant answer upfront. This reduces frustration and improves overall satisfaction with the information retrieval process.
-
Facet 4: Continuous Improvement
MLQs are continuously refined and improved through machine learning algorithms that analyze user feedback and interaction data. This ongoing learning process ensures that MLQs remain effective and accurate over time.
In summary, the example provided showcases the multifaceted benefits of MLQs in enhancing the accuracy, efficiency, and user experience of information retrieval systems.
Relevance to Main Topic
Most likely too questions (MLQs) play a pivotal role in information retrieval, as they are specifically designed to identify the most likely correct answer to a user’s query. This alignment with the main topic of information retrieval underscores the importance of MLQs in enhancing the effectiveness and accuracy of search results.
-
Facet 1: Improved Relevance
MLQs leverage statistical modeling and machine learning algorithms to analyze vast amounts of data, including historical search patterns, user behavior, and document content. By prioritizing the most relevant answer, MLQs ensure that users are presented with the most pertinent information that aligns with their query’s intent.
-
Facet 2: Enhanced Accuracy
The statistical and machine learning techniques employed in MLQs contribute to increased accuracy in identifying the most likely correct answer. MLQs continuously learn and adapt based on user feedback and interaction data, refining their models over time to provide more precise and reliable results.
-
Facet 3: Streamlined User Experience
MLQs streamline the user experience by minimizing the need to manually sift through numerous search results. By presenting the most likely correct answer prominently, MLQs save users time and effort, leading to greater satisfaction and productivity.
-
Facet 4: Foundation for Advanced Applications
MLQs serve as the foundation for advanced applications in information retrieval, such as personalized search results, conversational search assistants, and automatic question answering systems. Their ability to identify the most likely correct answer enables these applications to provide users with tailored and efficient information access experiences.
In summary, the relevance of MLQs to the main topic of information retrieval stems from their ability to enhance the effectiveness and accuracy of search results. By leveraging statistical modeling, machine learning algorithms, and continuous learning, MLQs play a critical role in improving the relevance, accuracy, user experience, and foundation for advanced applications in the field of information retrieval.
Frequently Asked Questions about Most Likely Too Questions (MLQs)
This section addresses common questions and misconceptions surrounding most likely too questions (MLQs), providing concise and informative answers.
Question 1: What are MLQs and how do they work?
MLQs are a specialized type of question employed in information retrieval systems to identify the most likely correct answer to a user’s query. They leverage statistical modeling and machine learning algorithms to analyze vast amounts of data and estimate the probability of each potential answer being the most relevant.
Question 2: How do MLQs contribute to the accuracy of search results?
MLQs enhance the accuracy of search results by prioritizing the most likely correct answer based on statistical analysis and machine learning techniques. These techniques consider factors such as historical search patterns, user behavior, and document content to identify the answer with the highest probability of being accurate.
Question 3: How do MLQs improve the user experience?
MLQs streamline the user experience by minimizing the need for manual sifting through numerous search results. By presenting the most likely correct answer prominently, MLQs save users time and effort, leading to increased satisfaction and productivity.
Question 4: What is the role of statistical modeling in MLQs?
Statistical modeling plays a fundamental role in MLQs by providing a quantitative framework for estimating the probability of each potential answer being the most relevant. These models analyze historical data and user behavior patterns to assign relevance scores to each answer.
Question 5: How do machine learning algorithms enhance MLQs?
Machine learning algorithms empower MLQs with the ability to learn and improve over time. These algorithms identify patterns and relationships in the data, allowing MLQs to refine their statistical models and enhance their accuracy in identifying the most likely correct answer.
Question 6: How are MLQs used in practical applications?
MLQs are widely employed in modern search engines, information retrieval systems, and other applications that require accurate and efficient retrieval of information. They are used to prioritize search results, provide personalized recommendations, and facilitate conversational search experiences.
In summary, MLQs are a valuable tool in information retrieval, enhancing the accuracy, efficiency, and user experience of search results through statistical modeling and machine learning techniques.
To learn more about MLQs and their applications, explore the following resources:
Tips for Utilizing Most Likely Too Questions (MLQs)
Most likely too questions (MLQs) are a powerful tool for enhancing the effectiveness and accuracy of information retrieval systems. Here are some tips to leverage MLQs effectively:
Tip 1: Leverage Historical Data and User Behavior Patterns
Gather and analyze historical data and user behavior patterns related to your target queries. This information will provide valuable insights for training MLQs and improving their accuracy.
Tip 2: Employ Machine Learning Algorithms
Incorporate machine learning algorithms into your MLQs to enable continuous learning and refinement over time. This will enhance the accuracy and adaptability of your MLQs.
Tip 3: Optimize Statistical Models
Fine-tune the statistical models used in your MLQs to ensure they accurately represent the probability distribution of potential answers. Regular evaluation and adjustment are key.
Tip 4: Prioritize User Intent
Design your MLQs to capture the user’s intent and context. Understanding the user’s information need will improve the relevance and usefulness of the most likely correct answer.
Tip 5: Continuously Monitor and Evaluate
Regularly monitor and evaluate the performance of your MLQs. Use metrics such as accuracy, precision, and user satisfaction to identify areas for improvement.
Tip 6: Consider Contextual Factors
Incorporate contextual factors, such as user location, device, and search history, into your MLQs to provide more personalized and relevant results.
Tip 7: Explore Advanced Applications
Explore advanced applications of MLQs, such as personalized search results, conversational search assistants, and automatic question answering systems, to enhance the user experience.
Summary
By following these tips, you can effectively utilize MLQs to improve the accuracy, efficiency, and user experience of your information retrieval system. Remember to prioritize data analysis, leverage machine learning algorithms, optimize statistical models, and continuously monitor and evaluate your MLQs for optimal performance.
Conclusion
Most likely too questions (MLQs) have emerged as a cornerstone of modern information retrieval systems, revolutionizing the way users access and interact with information. Their ability to identify the most likely correct answer to a user’s query, leveraging statistical modeling and machine learning algorithms, has significantly enhanced the accuracy, efficiency, and user experience of search results.
As the field of information retrieval continues to evolve, MLQs will undoubtedly play an even more pivotal role. By embracing advanced techniques, incorporating contextual factors, and exploring novel applications, we can harness the full potential of MLQs to empower users with the most relevant, accurate, and personalized information at their fingertips.
Youtube Video:
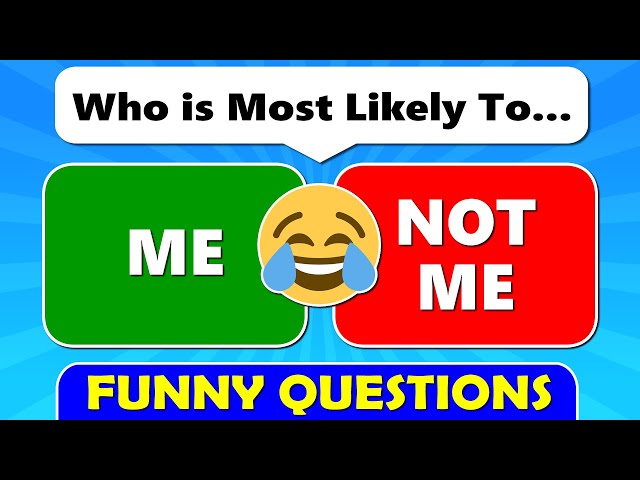